In the world of artificial intelligence (AI), data is the foundation upon which everything is built. Without high-quality data, AI algorithms cannot learn, evolve, or make accurate predictions. As AI becomes increasingly integrated into our lives and industries, why it is essential to ensure that the data used to train and fuel AI systems is accurate, reliable, and unbiased. Data quality in AI and, its impacts on AI systems. We will also discuss some best practices for improving data quality and the potential consequences of neglecting data quality in AI.
AI relies on data to learn and make decisions, and the quality of that data is crucial to the performance and accuracy of AI systems. Poor data quality can lead to biased and incorrect decisions, which can have serious consequences for individuals and society as a whole.
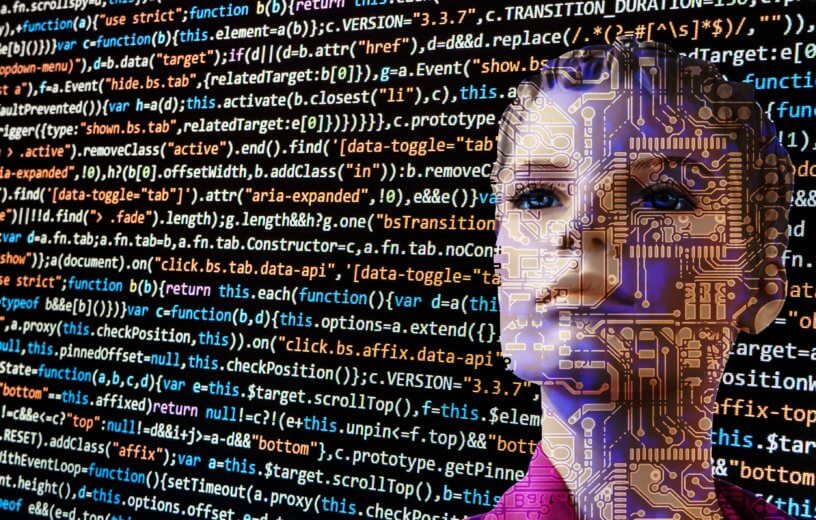
Effects of data quality on AI
Considering data quality in AI
Accurate and reliable data
AI systems must be trained with accurate and reliable data to ensure that the decisions made by the AI are correct. Incorrect or incomplete data can lead to inaccurate predictions or conclusions, which can be detrimental to individuals and organizations. For example, inaccurate medical data used to train an AI algorithm could result in incorrect diagnoses and treatments.
Unbiased data
AI systems must also be trained on unbiased data to ensure that they do not perpetuate or amplify existing biases or discrimination. Biased data can lead to biased decisions, which can have serious implications for individuals and society. For example, if an AI system is trained on data that contains gender or racial biases, it may perpetuate those biases in its decision-making.
Data cleaning and preprocessing
To ensure that data is accurate, reliable, and unbiased, it is essential to clean and preprocess data before using it to train AI systems. Data cleaning involves identifying and removing errors, duplications, and inconsistencies in the data, while data preprocessing involves transforming and standardizing the data to make it more usable for AI algorithms.
Consistent data
Consistency in data is important to ensure that data are comparable and can be analyzed over time. confusion and analytical errors might result from inconsistent data. For instance, if sales data is recorded differently in different regions, it becomes difficult to compare sales trends and analyze the reasons behind the differences.
Complete data
Incomplete data can lead to incomplete analysis and wrong conclusions. For example, imagine a marketing team analyzing customer behavior and demographics to design a marketing campaign. If the data is incomplete, the team may miss out on critical information, resulting in ineffective marketing campaigns and missed opportunities
Data governance
Data governance refers to the policies and procedures that ensure the quality, security, and privacy of data. It is essential to have proper data governance in place to ensure that data used to train AI systems is of high quality and complies with legal and ethical standards
Data security
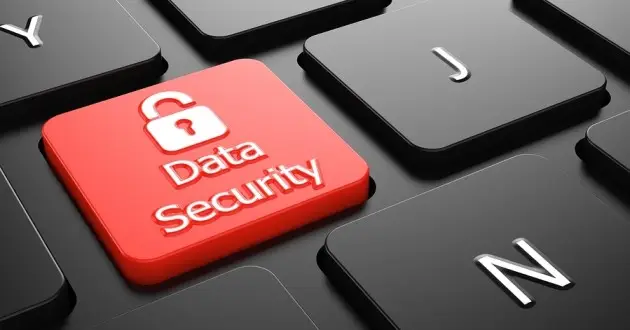
Data security is essential to protect sensitive information from unauthorized access, theft, and misuse. Data breaches can lead to financial losses, legal action, and damage to the company’s reputation. Data quality includes ensuring that data is secure and protected from unauthorized access.
Compliance with regulations
Many industries have regulations that require data to be accurate, complete, and secure. Failure to comply with these regulations can lead to fines, legal action, and damage to the company’s reputation. For example, the General Data Protection Regulation (GDPR) requires companies to ensure the accuracy and completeness of personal data. Companies that fail to comply with GDPR may face fines and legal action
The consequences of neglecting data quality in AI
The consequences of neglecting data quality in AI Consequences of neglecting data quality: Neglecting data quality in AI can have serious consequences, including inaccurate predictions, biased decisions, and legal and ethical violations. For example, in 2018, Amazon had to abandon an AI recruiting tool because it was biased against women due to the biased data used to train it.
Neglecting data quality in AI can have significant consequences, including:
Inaccurate predictions
If the data used to train AI algorithms is inaccurate or biased, the algorithms will make inaccurate predictions and draw incorrect conclusions.
Perpetuation of biases and discrimination
If the data used to train AI algorithms is biased, the algorithms will perpetuate that bias, potentially leading to discrimination and harm.
Biased algorithms
If data is not representative or diverse enough, the AI algorithm can produce biased results. For example, if facial recognition systems are trained only on images of white males, they may not be as accurate in identifying people from other genders, races, or ethnicities, which can lead to discriminatory outcomes.
Reduced trust
Poor data quality can also reduce trust in AI systems. If users do not trust the accuracy or reliability of the system, they may be hesitant to use it or rely on its output, which can limit its effectiveness.
Legal and financial consequences
If AI algorithms make decisions based on inaccurate or biased data, organizations may be held liable for the consequences. This can result in legal and financial consequences. For example, a company using an AI system for hiring decisions that discriminate against certain groups can face lawsuits and reputational damage
Erroneous decisions
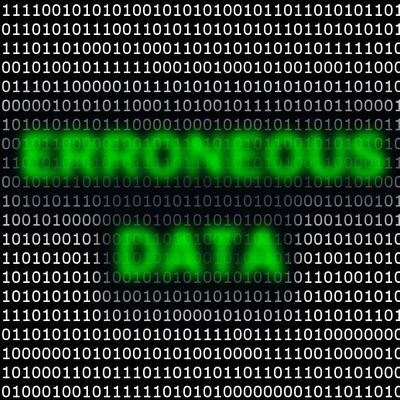
Poor quality data can lead to incorrect decisions by the AI system. For example, if a self-driving car’s algorithm is trained on poor-quality data, it may not be able to recognize certain objects or hazards on the road, leading to accidents.
we can say,
Data quality is critical to the performance, accuracy, and ethical use of AI systems. AI developers and organizations must prioritize data quality to ensure that AI systems are accurate, reliable, unbiased, and comply with legal and ethical standards.
To ensure that the data used to train AI algorithms is of high quality, there are several above-discussed best practices that organizations should follow. Organizations should invest in data quality programs and ensure that data is of high quality to avoid financial losses, missed opportunities, and damage to the company’s reputation
FAQs