In the rapidly evolving landscape of robotics and artificial intelligence, advancements continue to reshape the way robots interact with their environments and learn from them. One of the intriguing trends that has emerged is the transition from mere real-time adaptation to a more sophisticated concept – social learning in robot ecosystems. This transition marks a significant stride towards creating robots that not only respond to their surroundings but also actively learn from each other, akin to how humans learn from social interactions. This article delves into the fascinating journey from real-time adaptation to social learning in robot ecosystems and its implications for the future of robotics.
Real-Time Adaptation:
At its core, real-time adaptation in robotics involves the ability of robots to adjust their behavior based on immediate inputs from their environment. This concept, while essential for autonomous navigation and obstacle avoidance, represents a reactive approach to problem-solving. Real-time adaptation focuses on individual robot behavior without a larger context of shared knowledge or collaborative learning.
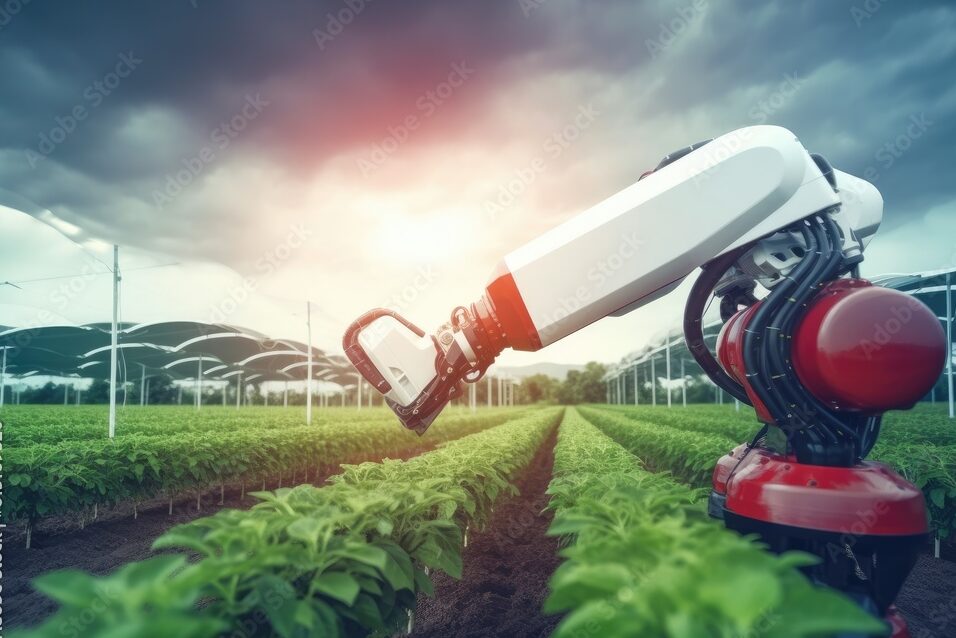
The Evolution: Social Learning in Robot Ecosystems:
As robotics technology has advanced, researchers have explored ways to elevate robot capabilities beyond simple real-time adaptation. Social learning, inspired by the human capacity to learn from each other’s experiences, has emerged as a promising avenue.
1. Knowledge Accumulation:
In a socially connected robot ecosystem, individual robots can pool their experiences and insights. This collaborative knowledge accumulation allows robots to collectively learn from a diverse range of situations and challenges, ultimately accelerating their learning curve.
2. Adaptive Strategies:
Social learning enables robots to adapt not only to specific situations but also to novel scenarios by drawing from the collective experiences of the ecosystem. This adaptive behavior goes beyond real-time adjustments and showcases a higher level of problem-solving skills.
3. Robustness and Resilience:
A robot ecosystem that practices social learning becomes more robust and resilient in the face of uncertainties. If one robot encounters an unexpected obstacle or challenge, its learnings can be quickly disseminated to the entire ecosystem, allowing others to proactively handle similar situations.
4. Learning Transfer:
Social learning facilitates the transfer of knowledge between robots, allowing a solution developed for one type of robot to benefit others. This cross-pollination of insights drives innovation and optimizes the overall performance of the ecosystem.
Challenges and Considerations:
1. Communication and Compatibility:
For robots to effectively engage in social learning, standardized communication protocols and compatible learning mechanisms are essential. Ensuring that robots can share and understand each other’s experiences is a technical challenge that researchers must address.
2. Privacy and Security:
Sharing experiences among robots could potentially raise concerns about data privacy and security. Striking a balance between collaborative learning and protecting sensitive information is crucial.
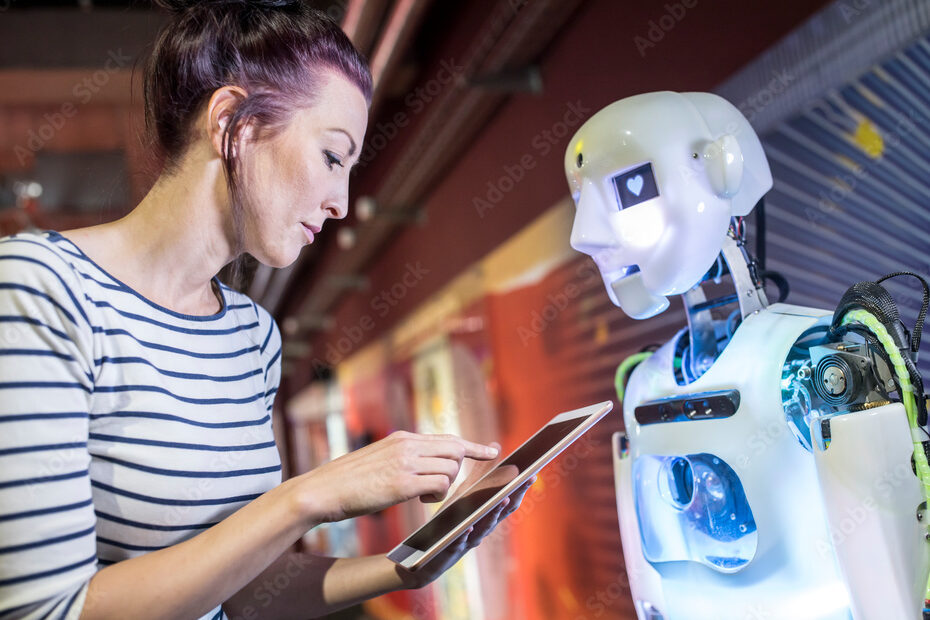
3. Scalability:
As the number of robots in an ecosystem grows, managing and coordinating their interactions for effective social learning becomes more complex. Scalability is a significant challenge that must be addressed to ensure the efficient functioning of large robot ecosystems.
4. Human Interaction:
Integrating robots into human environments and interactions presents challenges in terms of social norms, behaviors, and expectations. Robots must learn to navigate these social dynamics while maintaining their own learning and adaptability.
5. Resource Constraints:
Robots often operate within resource-constrained environments, such as limited processing power or energy. Balancing the demands of social learning with these constraints requires innovative solutions in resource management and allocation.
Conclusion
The transition from real-time adaptation to social learning in robot ecosystems represents a remarkable advancement in robotics. While challenges remain, the potential benefits, including enhanced collaboration, robustness, and adaptability, make this journey worth pursuing. By embracing responsible development and ethical considerations, we are poised to create a future where robots and humans coexist harmoniously, shaping a more efficient and innovative world. The road ahead is exciting, and the possibilities are vast.